Depending on how you view it, there is either a lot of talk of “synthetic data”…
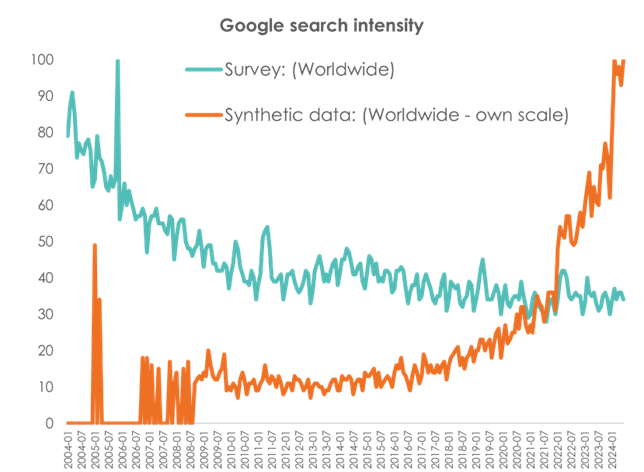
…or it’s a niche topic only of interest to the nerds.
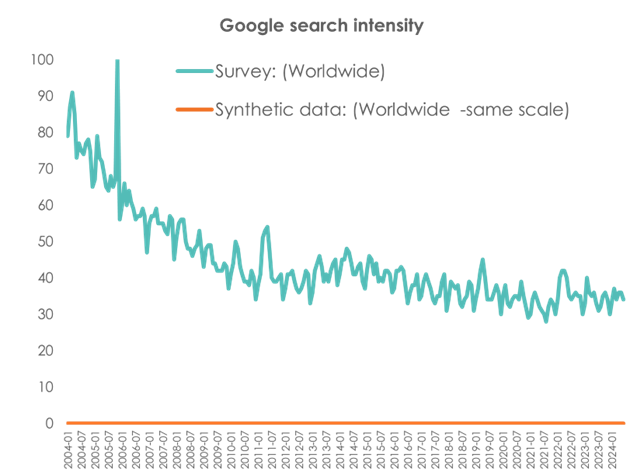
At Tapestry we have a lot of nerds. To us, therefore, it feels like a real thing – or a thing that will become increasingly more real over the next few months and years.
But what is “synthetic data” anyway? Most simply, synthetic data is information that is artificially generated rather than produced by real-world events.
The market research industry has been using synthetic data for years. For example:
- Dummy data to check survey routing.
- Fusion and/or imputation to join two surveys up or estimate what a respondent would have said if asked.
- Techniques such as agent-based modelling (ABM), some ways of using Bayesian statistical analysis and even some conjoint extensions (e.g. extending a price curve beyond what was tested) also creep into this space.
- Some people also say the increasingly popular MRP election polling is essentially synthetic data, as it is extending observed data into non-observed spaces.
Recently, however, the term has been reframed almost entirely around generative AI. Most typically ‘teaching’ an AI model to respond to questions in the manner of a ‘typical’ respondent or typology. The two most common uses are:
- Creating a chatbot-like interface that allows you to ask ‘questions’ of a given segment or typology (e.g. we do a segmentation and then create a synthetic ‘respondent’ for each segment that can be interacted with as if you were interviewing them).
- Creating an essentially quantitative ‘sample’ and getting it to answer a survey or respond to concepts, creative or brands.
This may feel like a threat to more traditional survey-based research. After all, this is essentially ‘teaching’ an AI model to behave like a survey. This may sound like the apocalypse for anyone that does lots of survey research. We can currently cling to the fact that generative AI models – usually based on large language models – aren’t great at numbers. This won’t be the case forever. It might not even be the case next week.
At this point I should acknowledge my inherent bias: I, and all of us at Tapestry, do a lot of survey-based research. This can make it very tempting to either defend survey research to the death or throw large amounts of shade on the very concept of synthetic data. Ideally both, in fact, just to be sure.
To go down this path would be very comforting. It is not, however, a path we should take. Some of us are old enough to remember the big switch from face-to-face polling to online research. There were many arguments in favour of the status-quo, with much talk of stratified sample frameworks being far superior to inadequate quota samples, and how the absence of an interviewer would lead to far less insight and rigour.
In the end, of course, online won and won quickly. It was faster, cheaper and provided results that were hard to argue with. It feels as if we are at the same point in the development of synthetic data right now. We can dismiss it and defend the status-quo, or we can work hard to make it as good as it can possibly be.
This means putting the data back into synthetic data.
Really, synthetic data done well would be a brilliant thing for survey-based research. It will mean our data is used far more effectively than it is right now. Anyone who works with survey data will often have that nagging concern that they’ve only scratched the surface of what you could do with the numbers you have. You’ll also often find yourself doing a project that feels so similar to something you’ve done before, often for the very same client, that you could write the debrief without looking at a single piece of data.
So let’s not fight the concept of synthetic data. Instead, let’s ask ourselves what we want it to be: Do we want it to be social-listening with better analytical capabilities? Or do we want it to be built on more deliberative, better designed data, interpreted brilliantly and updated regularly with similarly good quality data?
If we can make the case that the data part of synthetic data is the most important part, we may find ourselves doing the job we always claim market research to be. We’ll do really good research, make use of the most cutting-edge tools to analyse and join that data up – including to all sorts of other information sources – and spend much more time thinking about what it means for our clients.
We’ll also be better informed about the gaps in our knowledge and the additional research we need to do to fill those gaps. We’ll do far fewer of those studies that feel like re-treading the same old ground and we’ll spend our time testing a handful of really solid hypothesis rather than chucking everything in and panning for gold once fieldwork has finished.
Essentially, we’ll learn more and we’ll keep learning from everything we do, rather than relying on institutional knowledge to keep the memory of past projects alive. Our research really will live on, which many of us say but few of us really achieve on a regular basis.
In short, keep questioning how synthetic data is being defined. Keep questioning how it is being used. But use it, test it, improve it and, most of all, work as hard as possible to redefine it.
We’re only at the beginning of this adventure at Tapestry – as many of us are finding, the traditional ways of doing things don’t mesh easily with the new world – but it’s an adventure we’re really excited about.